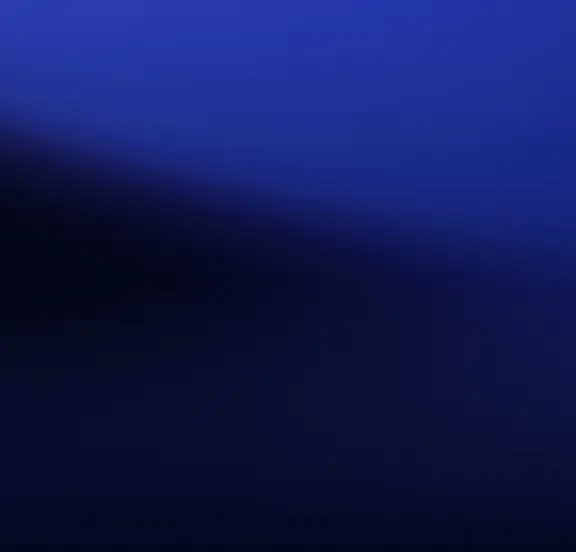
August 28, 2023 5 minutes read
Artificial Humans: AI Genome Generator
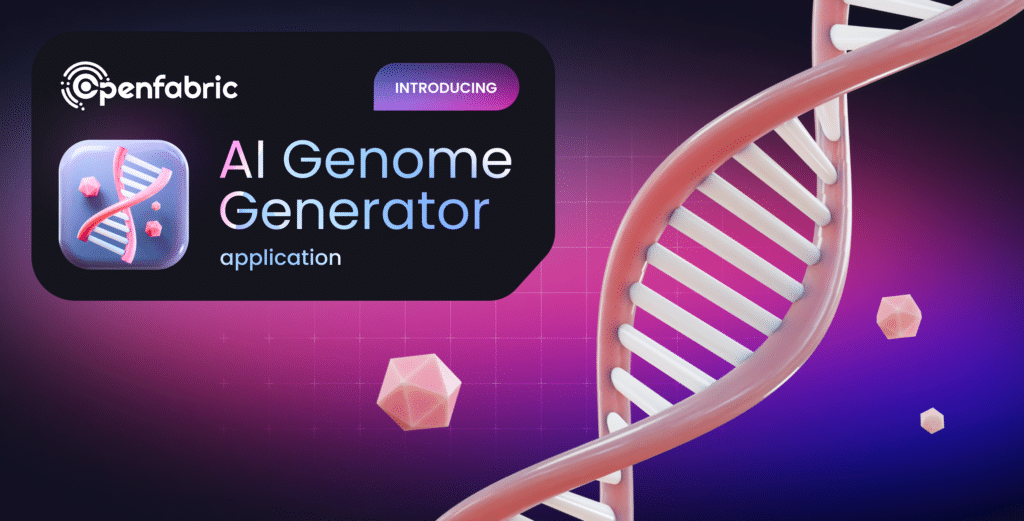
We can say it now without a doubt – this year in AI belongs to generative models. Spanning multiple disciplines and boasting exciting results, it is hard to pinpoint single other technology that can compare. However the limelight has been taken by the likes of image and text generation, making it easy to oversee other revolutionary use cases handled by generative AI. And what if we tell you that the power of this technology can be used to generate the very fabric of our biology – our own DNA.
Openfabric AI took upon the task to turn eyes towards more unusual examples and this one is definitely one of them. Thanks to the ability of generative models to comprehend complex data structures without strict supervision, researchers have proposed to use them as producers of artificial genomic data that could be used by researchers without the need for resource-intensive data gathering, as well as to evade potential privacy risks that stem from using human genome.
Following the amazing work presented in this article, we created an easy-to-use application that gives you an opportunity to generate an artificial genome and visualize its components. In order to achieve this we have trained the WGAN model to generate single nucleotide polymorphisms (SNPs) in the DNA sequence to predict the 3D structure of proteins that could be produced from the generated, novel DNA sequence. Letâs go through an overview of these components to better understand the inner workings of this application.
SNPs can help us predict a multitude of individual differences, including drug reactions and risks of disease development to name a few. These polymorphisms appear on the very basic level of the genetic structure, being simply replacements of single nucleotides (one of the four letters of the DNA) in comparison to the reference genome. The WGAN model was trained on a set of known SNPs located in chromosome 15, and is able to generate a plausible sequence of these SNPs, which we were able to map onto the reference code of this chromosome.
In order to give a visual representation to this sequence, we first transcribe and translate it from DNA, through RNA to amino acid sequence, which then in turn is used to create the very building blocks of our organism – proteins Since we know both of the transcription and translation mechanisms, we are able to map the specific nucleotides from the DNA to their corresponding nucleotides in RNA, and then recognize known codons in RNA which are translated to amino acids. Codons are simply triplets of nucleotides that form the basis of amino acids. Most of these triplets decide which amino acid to create, except for three stop codons, which terminate the translation process for a given sequence. That way, at the end of this process, we arrive at an amino acid sequence, the blueprint for proteins.Â
But how do we visualize a protein? Simply presenting a sequence of letters is not sufficient. What does a specific protein sequence potentially look like? This question is far from trivial, as folding of proteins is a complicated process. In order for the protein to actually work, it has to be folded into a 3D structure, as it is then that it gains its biological function.
Thanks to tremendous efforts of researchers we were able to use the ESMFold large protein language model to try predicting their 3D structure. The ingenuity of this model comes from the fact that it performs this prediction solely based on a sequence of amino acids presented in text form. This is why we are able to utilize its full potential in order to visualize proteins, as the genome generation process operates simply on numerical positions and letters representing nucleotides. Using the predicted 3D structure, we come back to the knowledge of SNP positions, adding specific markers on the folded protein to annotate where exactly the generated nucleotides went and what amino acid they translated to. The generated output we provide is interactive and available for download in the generally accepted .pdb format.
Generate genome
As the ESMFold in itself is a highly useful model, we also provide an option to simply predict the 3D structure of a given DNA, RNA or amino acid sequence.
Visualize DNA/ RNA/Amino Acid sequence
What is more, using the knowledge of SNPs positions in our newly generated chromosome, we know which genes and subsequences contain them – therefore, we are able to pinpoint their placements in the 3D representation. Thanks to publicly available annotated genome data we are able to extract specific genes from our artificial chromosome 15 and visualize these genes in a form of a 3D convoluted structure, annotating exact positions in which the SNPs were generated. Since the frequency of SNPs is rare, we added an option to predict a 3D structure of a subsequence that contains the largest amount of generated SNPs. All these representations are annotated with an amino acid name, which was translated from the triplet that contained the SNP.
Last but not least, we provide the generated chromosome data, SNP positions and the generated 3D structure as downloadable files. Therefore, if you want to try working with them on your own, itâs right there for you to get these files without a hassle.
This application is barely scratching the surface of what can be achieved with this technology in terms of aiding genetic and molecular research. Fostering development of such tools can speed up research that aims at improving our lives and well-being. Hopefully this use case will spark interest and prove great potential in generative AI in deeply scientific disciplines.
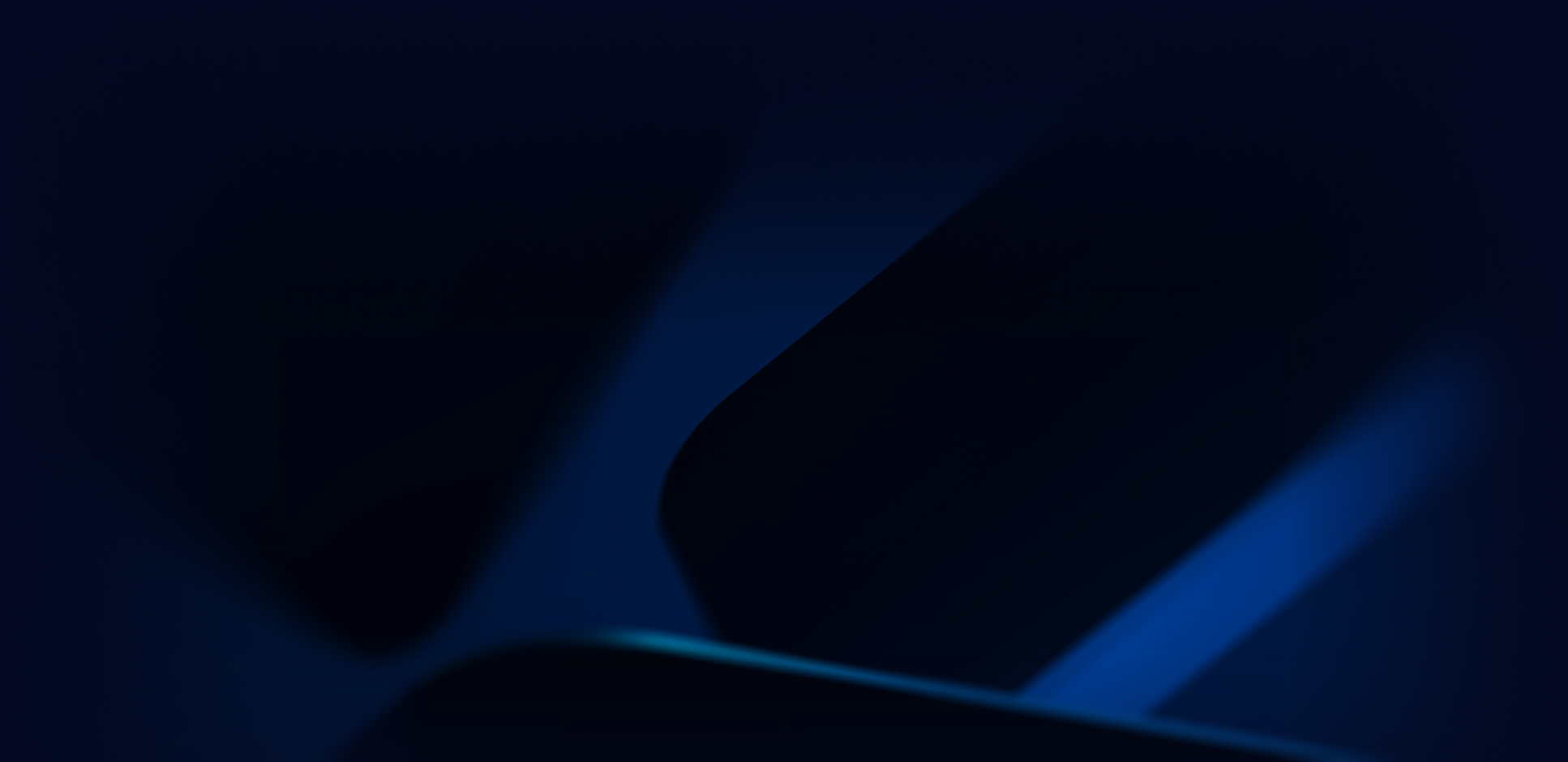